Let's Chat: AI in Healthcare
Q&A Matthew Murphy, Senior Director, Strategy and Performance and Chief Data Officer, Nova Scotia Health Authority about his keynote session on Navigating the AI-Powered Healthcare Revolution: Solutions, Challenges, and Stakeholder Perspectives.
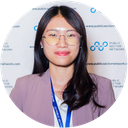
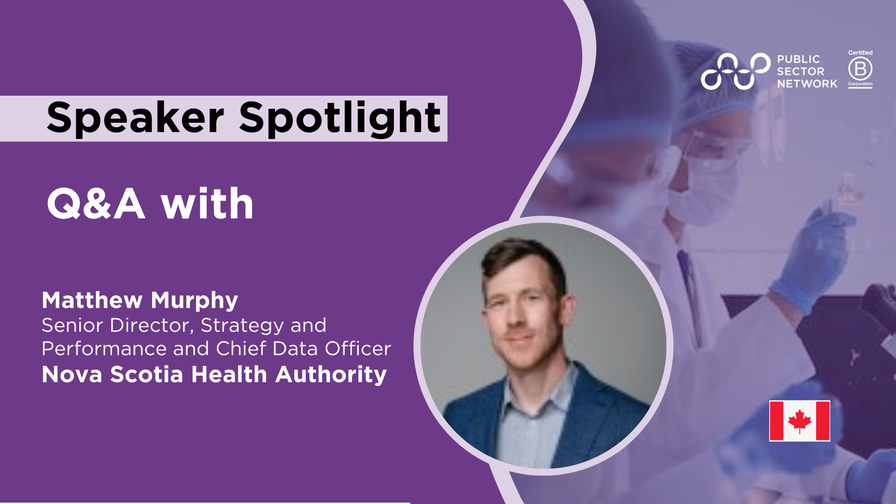
As our annual Healthcare Roadshow is approaching, we had the opportunity to interview Matthew Murphy, Senior Director, Strategy and Performance and Chief Data Officer, Nova Scotia Health Authority about his keynote session.
Q1: Among all the hot topics and trends in healthcare, why did you choose to talk about AI in your session?
AI is probably the most frequently discussed topic right now. Every conference, every vendor pitch, they all incorporate some aspect of how AI will improve our healthcare system. Either by improving patients experience, patient outcome, or through backend operations to support efficiency and effectiveness.
Within Nova Scotia we are far from immune to this trend and in fact are actively working to build an AI Centre of Excellence. It is an effort led by Dr. Gail Tomblin-Murphy, VP of Research and Innovation with Nova Scotia Health and will build upon the many exciting examples of AI at play in NS right now. That is another reason I wanted to speak on this topic. Many people view AI as this alien technology, or something to be feared. They picture robots replacing people or something from Terminator when in fact AI is ubiquitous and is already all around us making healthcare safer and more effective. We DO actually have robots, but they don’t replace humans, they augment their surgical prowess and help us perform higher quality surgeries to optimize patient outcomes. We also use AI to predict Emergency Department wait times, optimize surgical schedules and predict hospital overcrowding. We have research groups who are working with AI to detect cancer and other groups who are working to automate workflows and introduce chatbots. I wanted to talk about AI to help dispel some of the myths, highlight some of the amazing opportunities, and also discuss the need for effective governance and training to minimize bias and ensure ethical deployment.
Q2: Can you provide specific examples of how machine learning is currently being applied in healthcare to enhance patient outcomes?
Within Nova Scotia Health some examples would include:
- Overcapacity (surg) predictions that support proactive decision making to prevent overcapacity situations which can have negative impact on patient outcome and experience.
Emergency Department Wait Time Predictions to improve patient experience
Surgical Robots to improve the quality of surgical procedures thus improving patient outcomes
Surgical Scheduling Optimization which ensures we maximize surgical volumes and thus minimize wait times for individuals which has been shown to improve patient outcomes.
Diagnostic Imaging – this is not active within Nova Scotia but is at play in the healthcare space and has been shown to be an effective means of early cancer detection and diagnosis.
Early warning system that predict future patient decompensation and allow for early intervention to reduce both morbidity and mortality
Q3: How do these machine learning applications contribute to the overall efficiency and effectiveness of healthcare delivery?
I think there are myriad examples of how ML Ops contribute to increases in efficiency and effectiveness of healthcare delivery. Some specific areas that receive a lot of attention are:
Predictive Analytics: Machine learning algorithms can predict patient outcomes and identify patients at high risk of complications or readmissions. This allows healthcare providers to allocate resources more effectively, prioritize care for high-risk patients, and intervene proactively to prevent adverse events.
- Healthcare Operations Optimization: Machine learning techniques can optimize healthcare operations by analyzing patient flow, resource utilization, and scheduling processes. By identifying inefficiencies and bottlenecks, we can streamline operations, reduce waiting times, and improve overall patient experiences and outcomes.
- Diagnostic Imaging: Machine learning models can analyze medical images such as X-rays, MRIs, and CT scans to assist radiologists in detecting abnormalities and making more accurate diagnoses. Automated image analysis can speed up the diagnostic process and improve the accuracy of diagnoses.
- Early Disease Detection: Machine learning models can analyze patient data to identify early signs of diseases such as cancer, diabetes, and cardiovascular diseases. Early detection enables healthcare providers to intervene sooner, potentially improving patient outcomes and reducing treatment costs.
- Personalized Medicine: Machine learning algorithms can analyze large datasets, including patient health records, genomic data, and medical images, to identify patterns and tailor treatments to individual patients. This personalized approach can lead to more effective treatments with fewer side effects.
Q4: How do you ensure that the use of AI in healthcare maintains a balance between innovation and patient privacy/security?
Ensuring that the use of AI in healthcare maintains a balance between innovation and patient privacy/security requires a multifaceted approach that integrates technological safeguards, ethical considerations, and transparent communication.
Engaging patients, healthcare professionals, policymakers, regulators, and other stakeholders in the development, implementation, and evaluation of AI-driven healthcare solutions fosters shared responsibility and accountability. Collaboration across diverse stakeholders helps identify and address emerging challenges, promote best practices, and ensure that patient interests remain paramount. Keeping the patient interest front and centre is one of the most important aspects of this work, we must always ask ourselves who is benefiting from the work, who is at risk, and how have those risks been addressed.
Appropriate use of privacy-preserving AI techniques such as federated learning, differential privacy, and advanced encryption can help mitigate privacy risks associated with AI-driven healthcare applications. These techniques allow models to be trained and deployed without directly accessing sensitive patient data, thereby preserving privacy while enabling innovation.
Appropriate data governance is another necessity for balancing innovation with patient privacy and security. Establishing transparent data governance frameworks within healthcare organizations ensures that patient data is handled responsibly and ethically. These frameworks define policies, procedures, and accountability mechanisms for data access, sharing, and use, promoting trust and confidence among patients and stakeholders.
Obviously cyber plays a huge role too, ensuring that that all necessary security protocols have been implemented by a team with the proper technical background is essential to ensuring that patient data remains safe and secure.
By adopting these strategies and integrating them into the design, deployment, and governance of AI in healthcare, stakeholders can effectively balance innovation with patient privacy and security, fostering trust, and advancing the responsible use of AI technologies in healthcare delivery.
Q5: How can healthcare organizations effectively integrate these technologies into existing workflows without disrupting the overall healthcare delivery process?
Integrating AI technologies into existing workflows in healthcare organizations requires careful planning, collaboration, and adaptation to minimize disruptions. It is helpful to begin by conducting workflow analyses and needs assessments. Start by identifying areas where AI technologies can add value and address known challenges. Major disruptions often arise from trying to force a solution into an area that was already high functioning. Focusing on organizational pain points allows the implementation of AI solutions to garner those highly sought after “quick wins”. Solving a problem that staff are already facing is definitely a better approach than brining in new tech for an area that never flagged an issue in the first place.
Engaging with the frontline staff, clinicians, and leadership to understand their workflow challenges, pain points, and opportunities for improvement is the best way of surfacing the organizational pain points discussed above. Identifying the problems and developing solutions in a boardroom is never an effective strategy.
Honestly, avoiding disruptions with the implementation of AI solutions is no different than the adjustment of any workflow. Whether it is the implementation of new software, a new resource or a new position, you need to assess current state, understand the problem you are aiming to solve. Enact appropriate change management practices early on, ensure a collective understanding and willingness to move to the future state and provide consistent training and education. Where AI solutions are perhaps more novel than an email upgrade, extra focus on education and training is helpful, especially if there is distrust about AI.
Q6: How does the incorporation of AI and robotics align with a patient-centric approach in healthcare, and what benefits can patients expect to see from these technological advancements?
The incorporation of AI and robotics in healthcare aligns closely with a patient-centric approach by focusing on improving patient outcomes, enhancing the quality of care, and personalized treatment experiences. Tech advancements benefit patients in so many ways. A few examples, or areas of benefit would include:
- Personalized Treatment and Care Plans: As discussed above, AI algorithms can analyze vast amounts of patient data, including medical history, genetic information, and real-time physiological data, to develop personalized treatment and care plans tailored to each patient's unique needs and preferences. This patient-centric approach ensures that interventions are customized to optimize outcomes and enhance patient satisfaction.
- Precision Medicine and Predictive Analytics: AI-driven predictive analytics enable early detection of diseases, identification of risk factors, and prediction of treatment responses based on individual patient characteristics. By leveraging AI algorithms to analyze complex biological data, patients can expect to increasingly receive precision medicine approaches that optimize therapeutic outcomes while minimizing adverse effects and treatment failures.
- Enhanced Diagnosis and Decision Support: AI-powered diagnostic tools and decision support systems augment healthcare providers' clinical judgment by providing real-time insights, differential diagnoses, and treatment recommendations based on evidence-based guidelines and best practices. By facilitating accurate and timely diagnosis, AI technologies improve patient outcomes, reduce diagnostic errors, and streamline care delivery processes.
- Robotic-Assisted Surgery and Procedures: Robotics technology enhances surgical precision, dexterity, and efficiency, leading to better surgical outcomes, shorter recovery times, and reduced postoperative complications for patients. Robotic-assisted procedures enable minimally invasive interventions with smaller incisions, less pain, and faster recovery, resulting in improved patient comfort and satisfaction compared to traditional open surgeries.
Overall, the incorporation of AI and robotics in healthcare promotes a patient-centric approach by delivering personalized, efficient, and high-quality care that addresses patients' individual needs, preferences, and outcomes. Patients can expect to see tangible benefits from these technological advancements, including improved treatment outcomes, enhanced patient experience, greater access to care, and increased empowerment and engagement in managing their health and well-being.
Q7: Looking ahead, what emerging trends do you foresee in the intersection of AI, machine learning, robotics, and healthcare?
Looking ahead, several emerging trends are likely to shape the intersection of AI, machine learning, robotics, and healthcare, paving the way for transformative advancements in patient care, medical research, and healthcare delivery. Here are some key areas to watch:
- AI-Driven Drug Discovery and Development: AI and machine learning algorithms are revolutionizing the drug discovery process by accelerating the identification of novel drug candidates, predicting drug-target interactions, and optimizing drug design. AI-powered platforms enable virtual screening of compound libraries, de novo drug design, and drug repurposing, leading to faster, more cost-effective drug development pipelines and the discovery of breakthrough therapies for complex diseases.
- Precision Health and Predictive Analytics: Advances in AI and machine learning enable the integration of multi-omics data, digital biomarkers, and real-world evidence to deliver precision health approaches that tailor interventions to individuals' unique genetic, environmental, and lifestyle factors. Predictive analytics models leverage longitudinal patient data to anticipate disease risk, stratify patient populations, and optimize treatment strategies, enabling proactive, personalized healthcare delivery and disease prevention initiatives.
- Robotics-Assisted Healthcare Delivery: Robotics technologies are increasingly being integrated into healthcare delivery settings to automate routine tasks, assist healthcare providers in clinical procedures, and enhance patient care experiences. Robotic-assisted surgery, rehabilitation robotics, and robotic exoskeletons enable precise, minimally invasive interventions, personalized rehabilitation programs, and mobility assistance for patients with physical disabilities, improving functional outcomes and quality of life.
- AI-Powered Imaging and Diagnostics: AI algorithms are transforming medical imaging and diagnostics by improving the accuracy, speed, and efficiency of image interpretation, disease detection, and diagnostic decision-making. Deep learning models enable automated analysis of medical images, such as X-rays, MRIs, and CT scans, to identify abnormalities, classify diseases, and prioritize urgent cases, enabling early diagnosis and treatment initiation for better patient outcomes.
- Augmented Intelligence in Clinical Decision Support: Augmented intelligence solutions enhance clinical decision support systems by integrating AI-driven insights, evidence-based guidelines, and patient-specific data into clinicians' decision-making processes. These systems provide real-time alerts, recommendations, and predictive analytics to assist healthcare providers in diagnosing conditions, selecting treatment options, and optimizing care plans, improving clinical outcomes and patient safety.
Overall, the convergence of AI, machine learning, robotics, and healthcare is poised to revolutionize healthcare delivery, personalized medicine, and medical research, ushering in a new era of precision, efficiency, and patient-centered care. These emerging trends hold the promise of advancing healthcare outcomes, improving population health, and enhancing the overall well-being of patients and communities around the world.
Matt will be joining us in Halifax on May 3rd to share his thoughts and experiences in "Navigating the AI-Powered Healthcare Revolution: Solutions, Challenges, and Stakeholder Perspectives". Register today for an in-depth discussion with Matt on how AI, machine learning and other emerging technologies that are impacting Canada healthcare. Visit the event website here for more information: https://publicsectornetwork.com/event/healthcare-roadshow-canada/
- Related Event
- Healthcare Roadshow 2024
- Communities
- Technology & Transformation
- Region
-
Canada
Published by
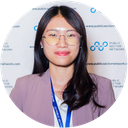
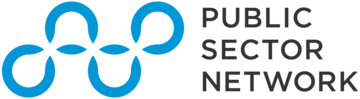
Most Popular